New Study Reveals AI Struggles with Reading Analogue Clocks and Calendar Date Calculations
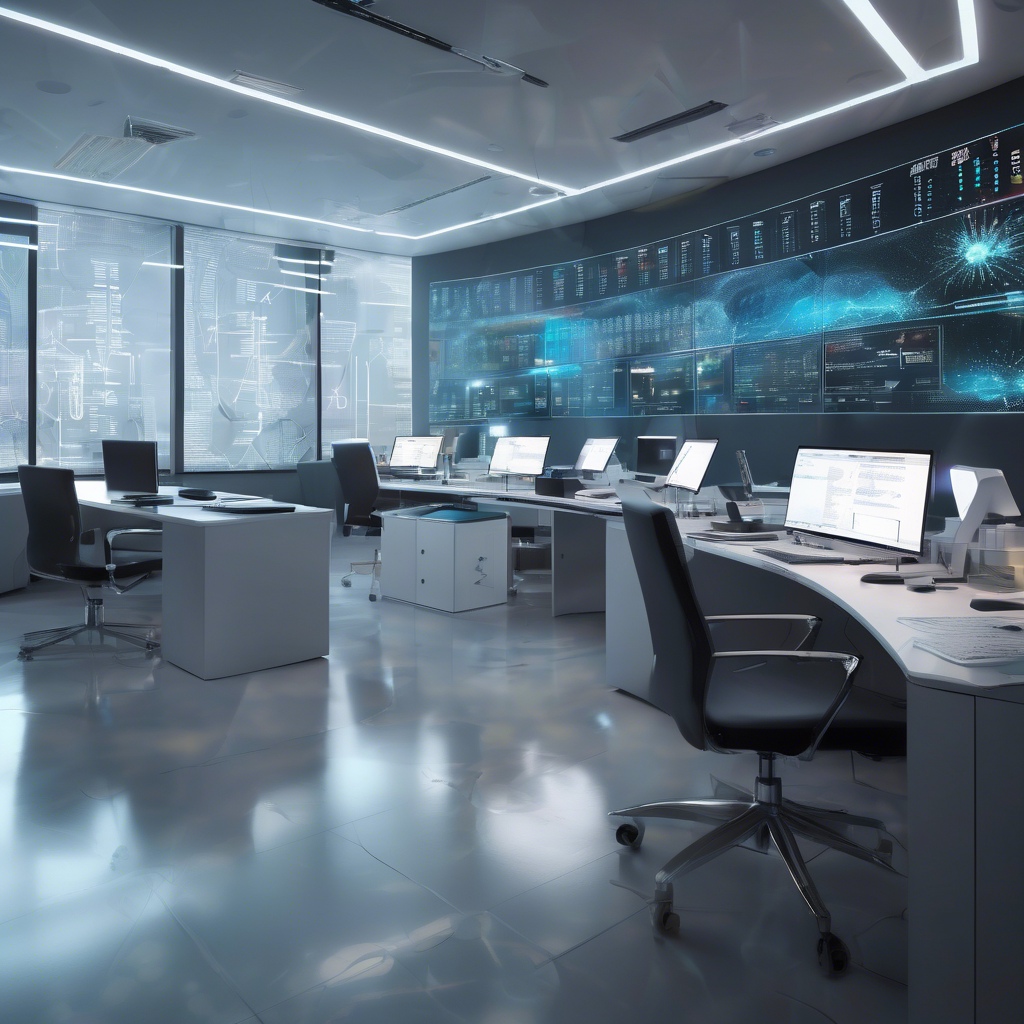
New research has identified a set of tasks that humans handle effortlessly but artificial intelligence (AI) struggles with—specifically reading analogue clocks and determining the day of the week for a given date. Although AI can generate code, images, human-like text, and even pass exams to varying degrees, it frequently misinterprets clock hand positions and fails basic calendar arithmetic. Presented at the 2025 International Conference on Learning Representations (ICLR) and published on the preprint server arXiv (not yet peer-reviewed), the study highlights significant gaps in AI’s ability to perform tasks humans master early in life. Lead author Rohit Saxena of the University of Edinburgh emphasized that these shortcomings must be addressed for AI to be effectively applied in time-sensitive and real-world contexts like scheduling, automation, and assistive technologies. The researchers tested various multimodal large language models (MLLMs)—including Meta’s Llama 3. 2-Vision, Anthropic’s Claude-3. 5 Sonnet, Google’s Gemini 2. 0, and OpenAI’s GPT-4o—using a custom dataset of clock and calendar images. The models failed to correctly identify clock times or determine weekdays for sample dates over half the time, with accuracy rates of only 38. 7% for clocks and 26. 3% for calendar tasks. Saxena explained that AI’s poor clock-reading stems from its lack of spatial reasoning—tasks requiring detection of overlapping hands, angle measurements, and interpreting diverse clock designs, such as Roman numerals or stylized dials. Recognizing an image as a clock is easier for AI than reading it accurately.
Similarly, despite arithmetic being fundamental to computing, large language models do not perform calculations via algorithms; instead, they predict outputs based on training data patterns. This leads to inconsistent and non-rule-based reasoning, explaining high failure rates on date-related arithmetic. This study adds to growing evidence that AI’s mode of “understanding” differs fundamentally from human cognition. AI excels when abundant training examples exist but struggles with abstract reasoning and generalization, especially on tasks mixing perception with precise logic. Furthermore, limited training data on rarer phenomena like leap years hampers performance, as AI fails to make necessary conceptual connections. The findings underscore the need for richer, targeted datasets and reevaluation of AI’s capability to integrate logical and spatial reasoning, highlighting risks of over-reliance on AI outputs in complex tasks. Saxena stressed the necessity of rigorous testing, fallback mechanisms, and often human oversight when AI is tasked with combining perception and exact reasoning.
Brief news summary
New research presented at the 2025 International Conference on Learning Representations highlights significant limitations in current AI models like Meta’s Llama 3.2-Vision, Anthropic’s Claude-3.5 Sonnet, Google’s Gemini 2.0, and OpenAI’s GPT-4o. Despite recent advancements, these models struggle with tasks that are straightforward for humans, such as reading analog clocks and determining weekdays from dates. The study found these models correctly interpreted clock times only 38.7% of the time and calendar dates just 26.3%, underscoring their reliance on pattern recognition rather than genuine reasoning abilities. Led by Rohit Saxena from the University of Edinburgh, the research reveals that while AI systems can identify objects accurately, they face notable challenges with complex spatial and logical reasoning tasks, especially involving uncommon events like leap years. The findings emphasize the need for new training approaches that integrate logical and spatial reasoning skills and warn against overdependence on AI for tasks requiring precise calculations. Ultimately, the study highlights fundamental differences between human cognition and AI pattern matching, advocating for comprehensive validation and human oversight in time-sensitive real-world applications.
AI-powered Lead Generation in Social Media
and Search Engines
Let AI take control and automatically generate leads for you!
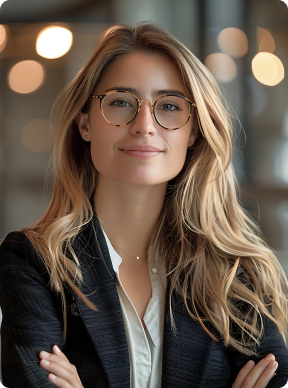
I'm your Content Manager, ready to handle your first test assignment
Learn how AI can help your business.
Let’s talk!
Hot news
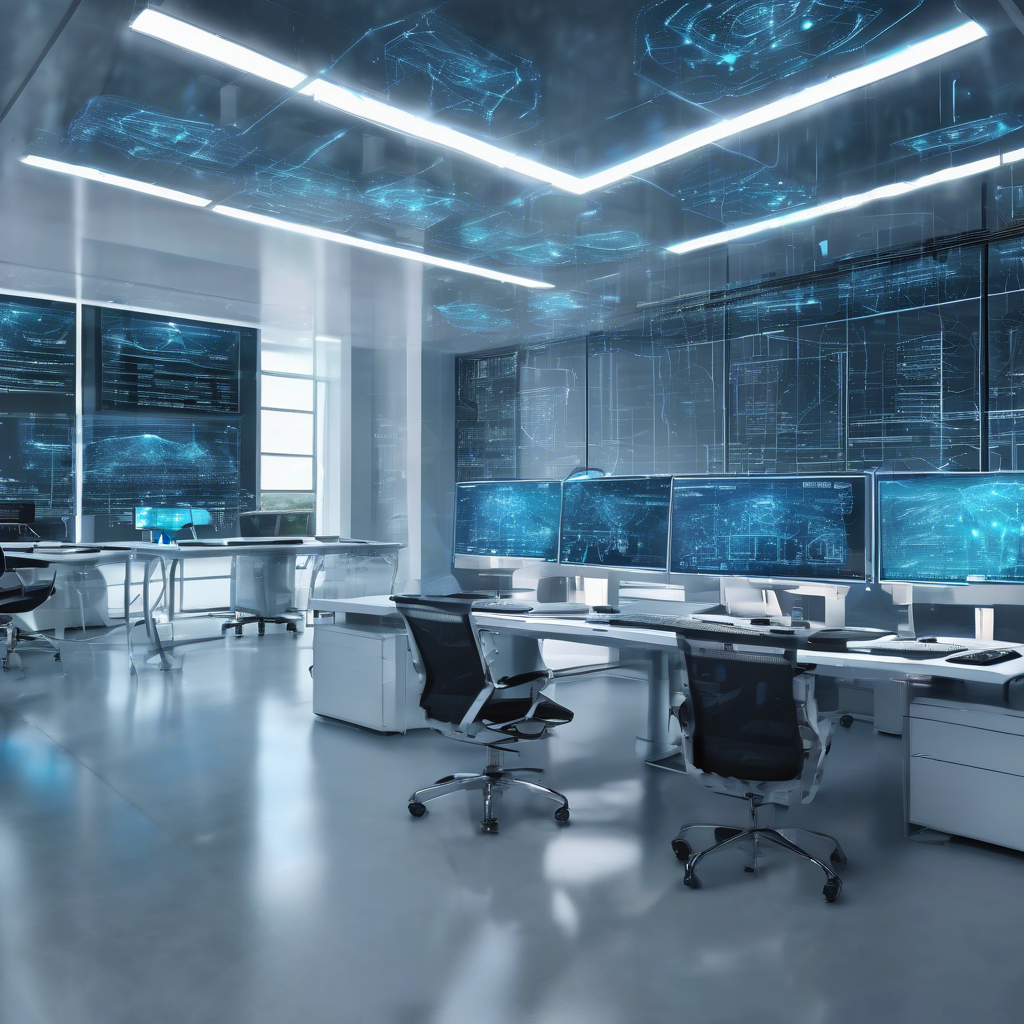
Ilya Sutskever Assumes Leadership of Safe Superin…
Ilya Sutskever has assumed leadership of Safe Superintelligence (SSI), the AI startup he founded in 2024.
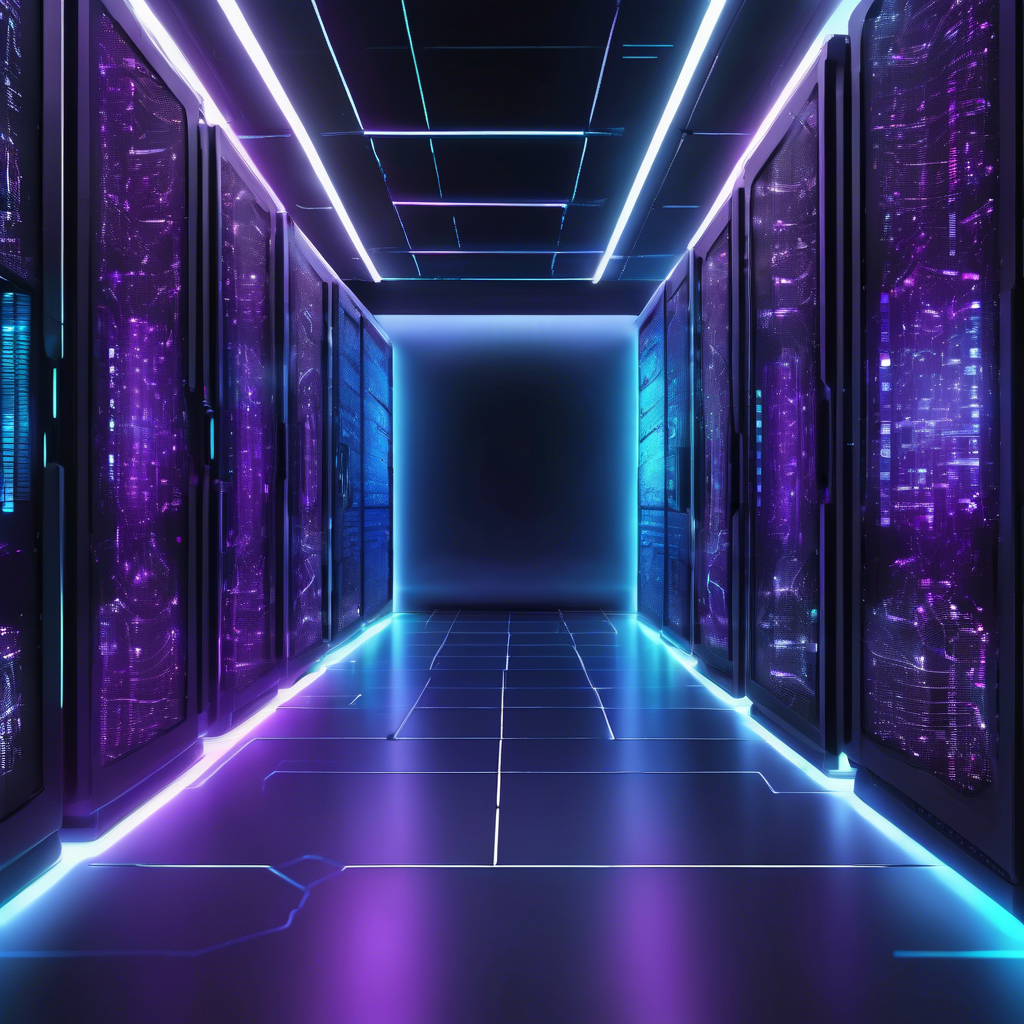
‘The world supercomputer’: Nexus activates final …
This segment is from the 0xResearch newsletter.
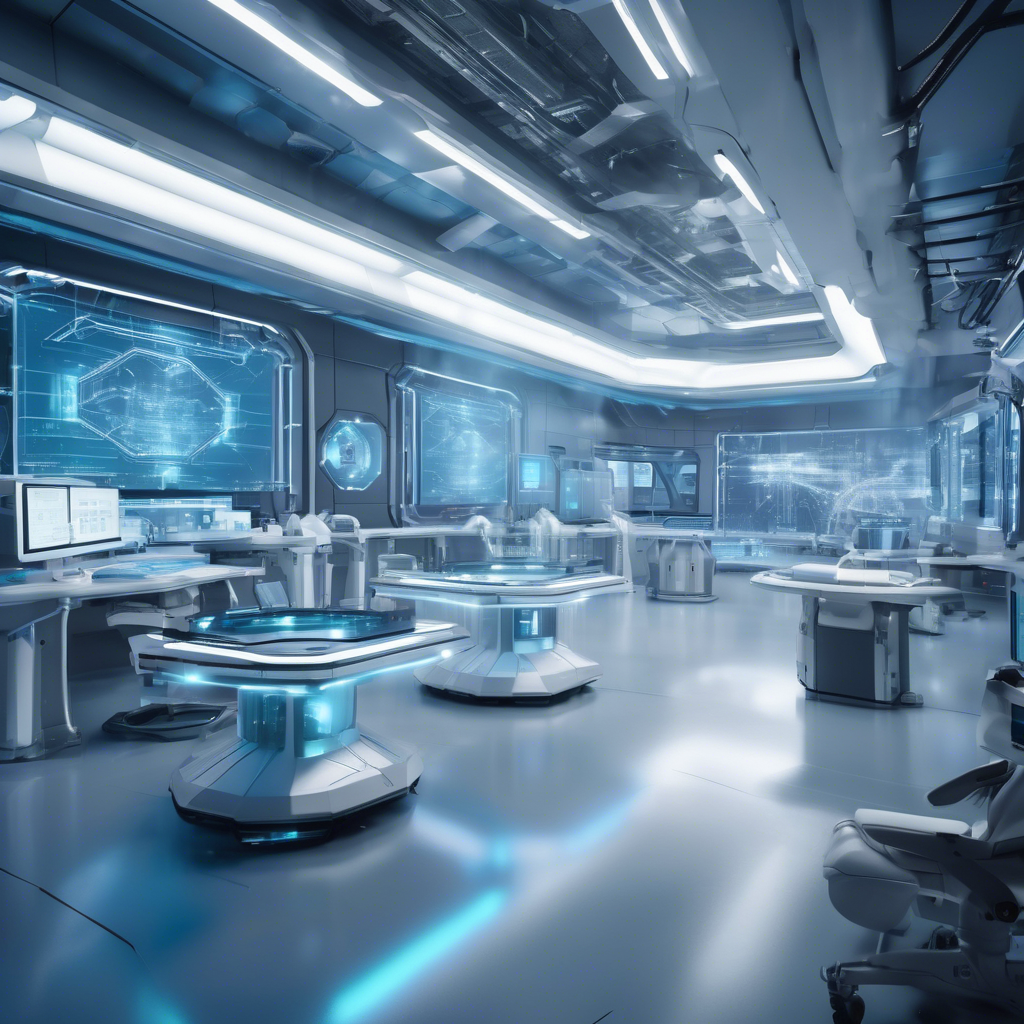
Tech Industry Collaborates with Pentagon to Enhan…
The collaboration between the U.S. technology sector and the Pentagon is intensifying amid rising global instability and the growing strategic relevance of artificial intelligence (AI).
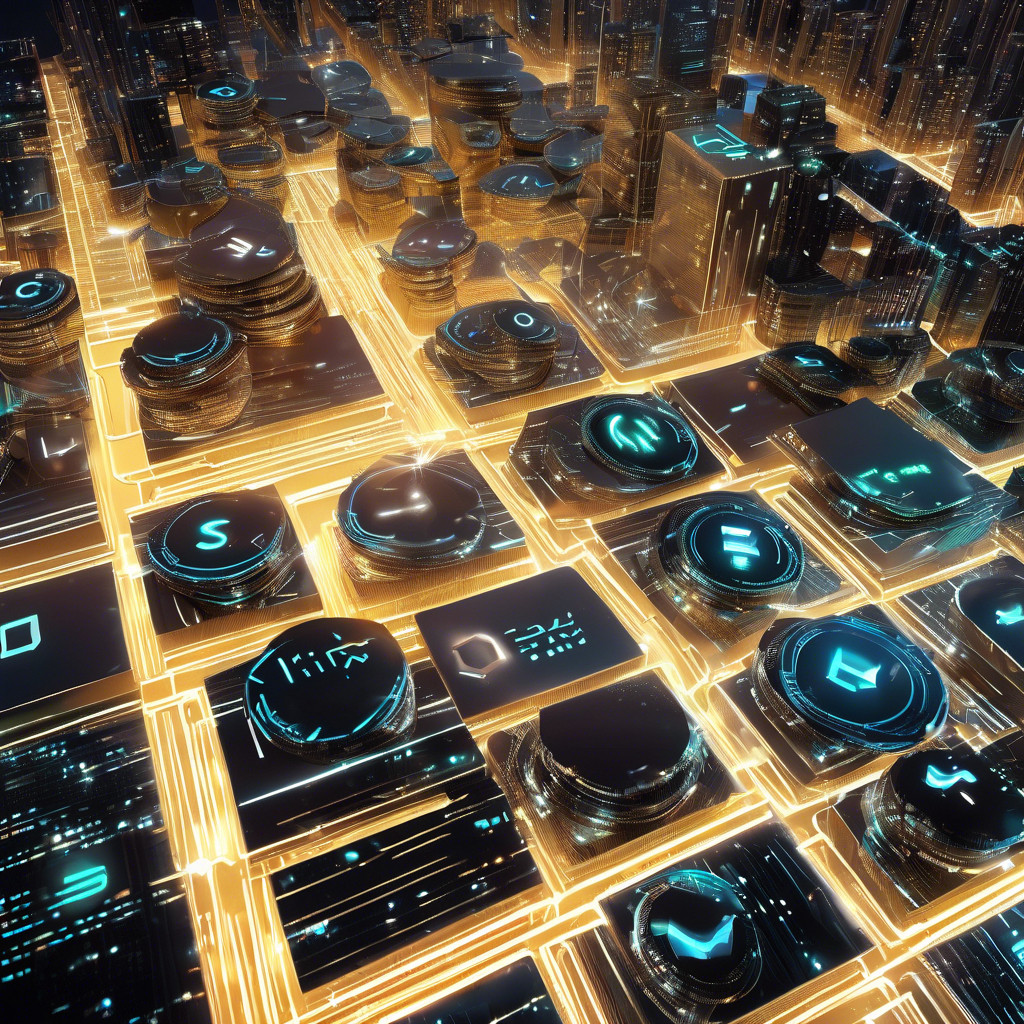
Stablecoins' Potential and Adoption Challenges
Stablecoins have been widely hailed as a transformative innovation for global payments, promising fast, low-cost, and transparent transactions that could revolutionize cross-border money transfers.
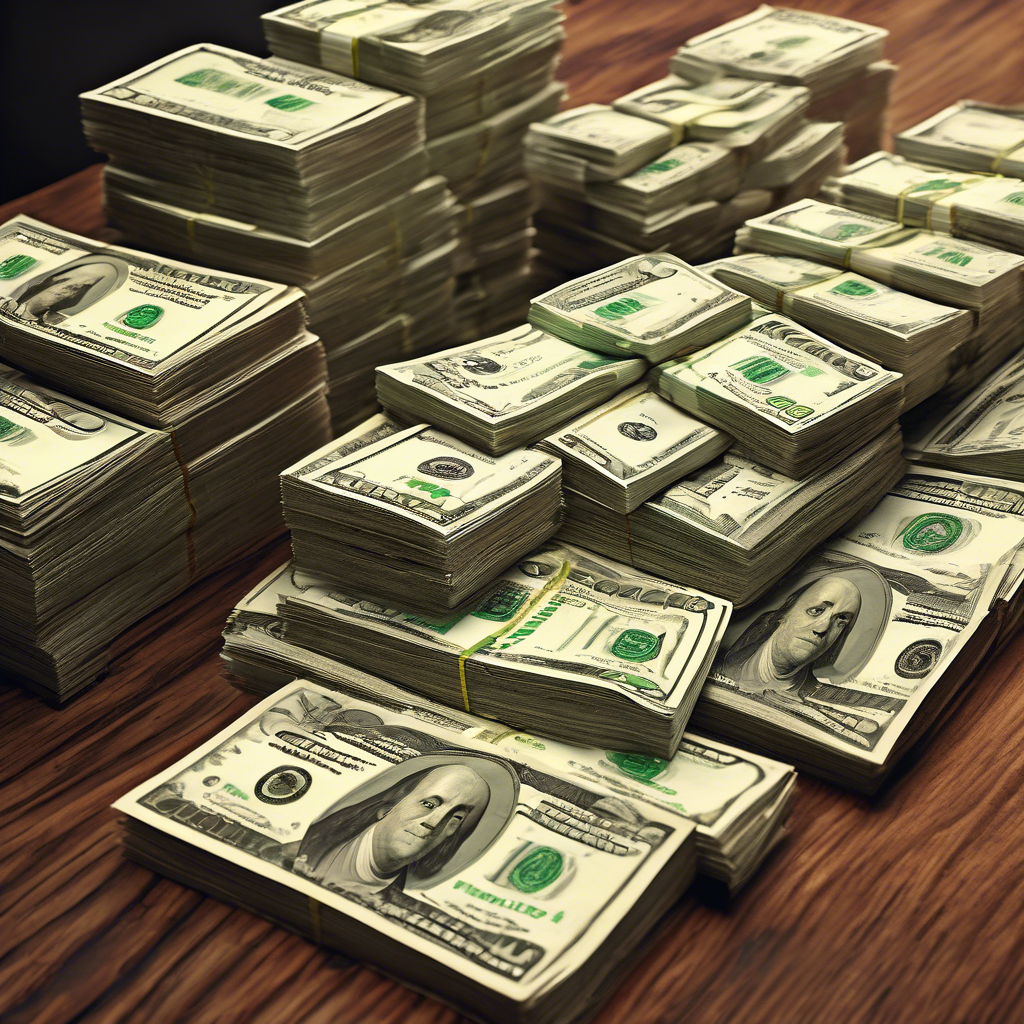
U.S. M2 Money Supply Reaches Nearly $22 Trillion
In May, the United States reached a significant economic milestone as its M2 money supply hit a record $21.94 trillion, marking a 4.5% increase from the previous year—the fastest growth rate in nearly three years.
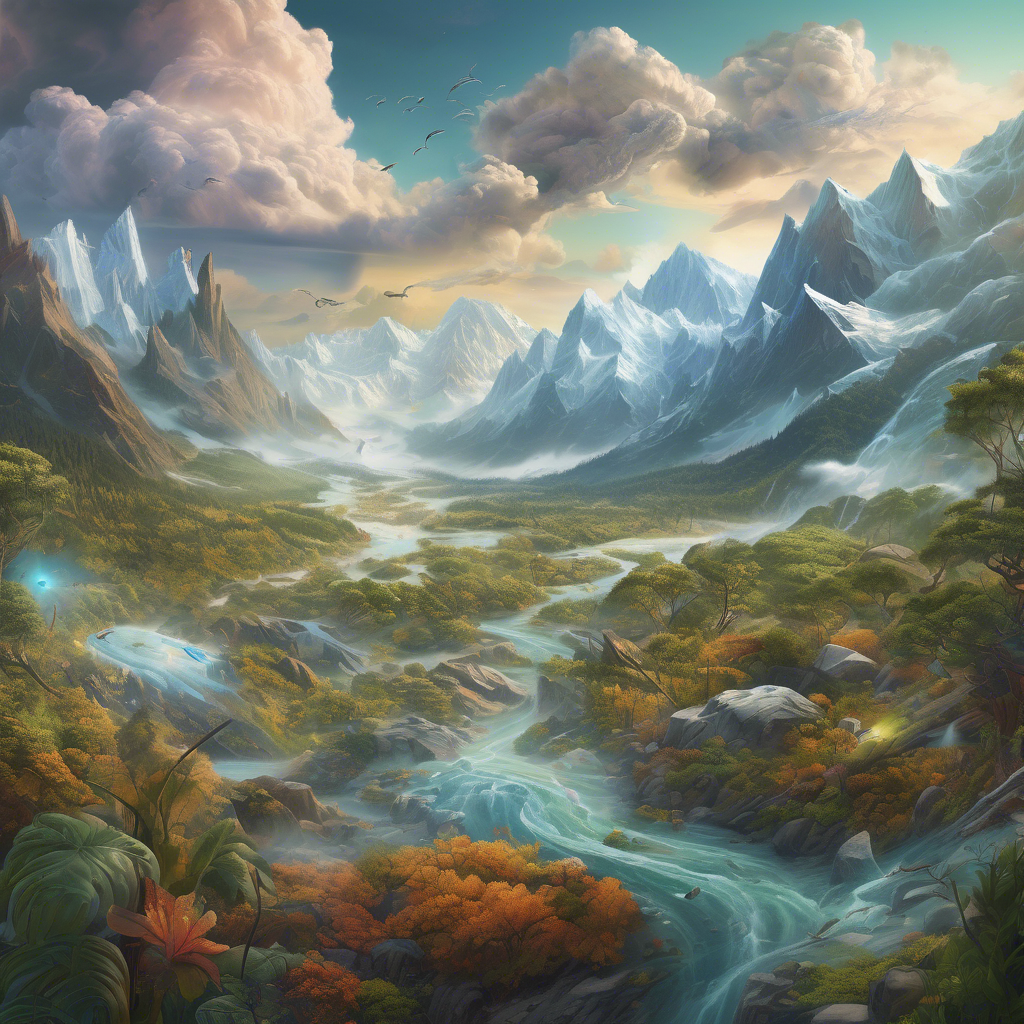
AI and Climate Change: Predicting Environmental S…
Scientists worldwide are increasingly utilizing artificial intelligence (AI) to enhance the understanding and prediction of climate change impacts on diverse ecosystems.
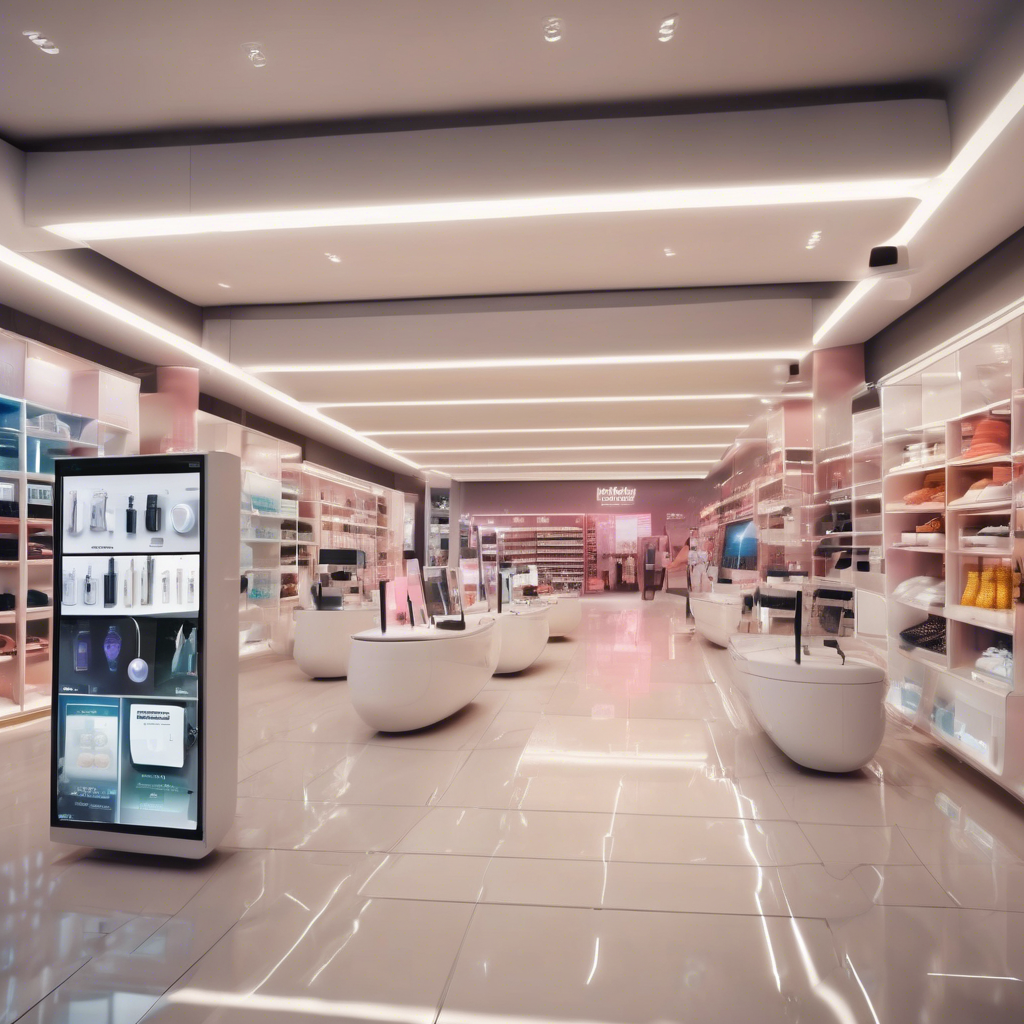
AI in Retail: Personalizing Customer Experiences
Artificial intelligence (AI) is profoundly transforming the retail industry, ushering in a new era of personalized shopping experiences tailored to the unique preferences and behaviors of individual consumers.