MIT Researchers Develop Periodic Table Unifying Over 20 Classical Machine Learning Algorithms
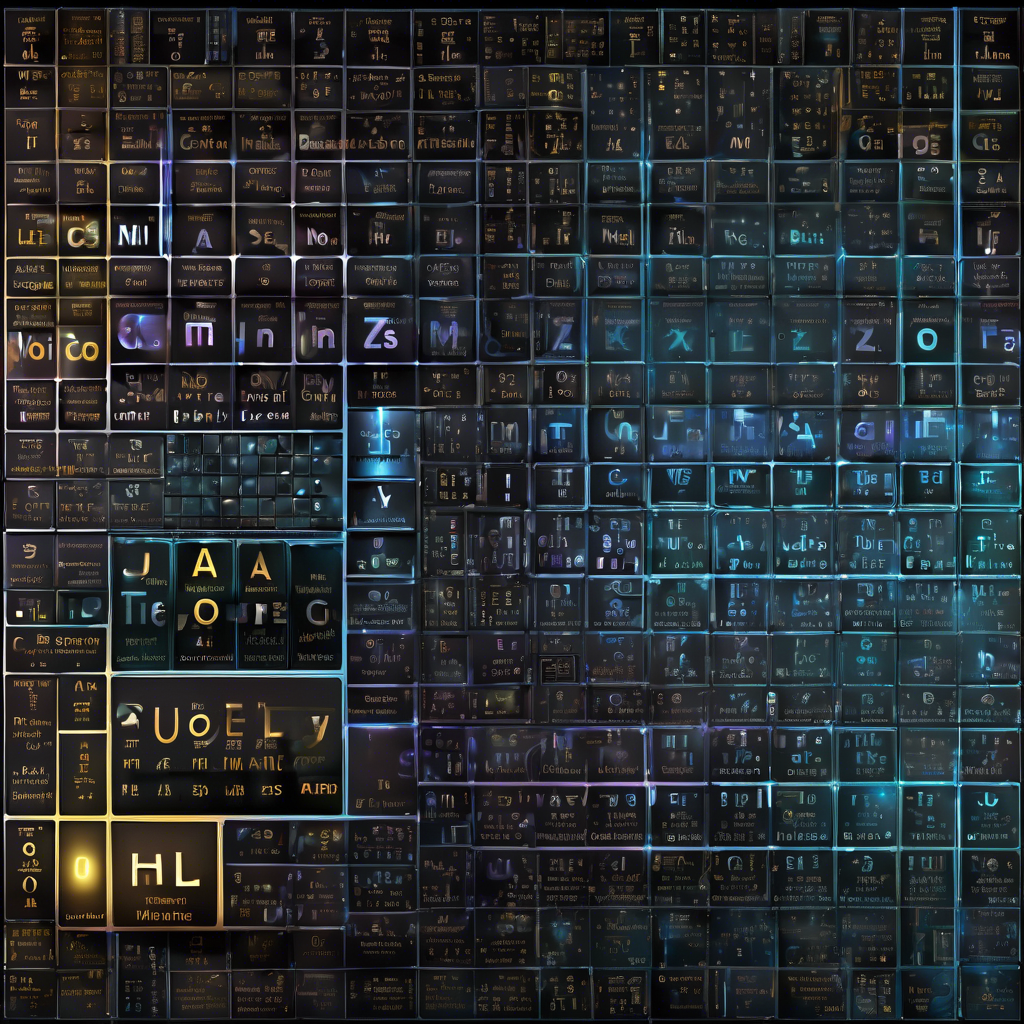
MIT researchers have developed a periodic table illustrating the connections among over 20 classical machine-learning algorithms, offering insights into how combining strategies from different methods can enhance existing AI models or inspire new ones. Using this framework, they created a new image-classification algorithm that improved performance by 8 percent over current leading techniques. The concept behind the table is that all these algorithms learn specific relationships between data points, with core mathematical principles shared across them despite differing implementations. From this, the team identified a unifying equation underlying many classical AI algorithms, which they used to reframe popular methods and organize them into a table based on the types of relationships each algorithm learns. Much like the chemical periodic table—with empty spaces predicting undiscovered elements—the machine-learning table also contains gaps suggesting where yet-to-be-invented algorithms may exist. This tool enables researchers to design new algorithms without re-deriving previous ideas, as noted by Shaden Alshammari, lead author and MIT graduate student, who emphasized that machine learning can now be viewed as a structured system ripe for exploration rather than guesswork. The paper’s authors include John Hershey (Google AI Perception), Axel Feldmann (MIT graduate student), William Freeman (MIT professor and CSAIL member), and senior author Mark Hamilton (MIT graduate student and Microsoft engineering manager). Their work will be presented at the International Conference on Learning Representations. The discovery of the unifying equation was serendipitous. Alshammari initially studied clustering algorithms that group similar images and noticed similarities to contrastive learning methods. Investigating further, she found both could be described by the same underlying equation.
As Hamilton recalls, once they uncovered this connection, they incorporated nearly every classical method they tested into this unified framework. Named information contrastive learning (I-Con), the framework demonstrates how various algorithms—from spam detection classifiers to deep learning models powering large language models—can be interpreted through this equation. The equation captures how algorithms approximate relationships between real data points by minimizing deviations from actual connections within training data. To categorize these algorithms, the researchers structured I-Con into a periodic table that sorts methods based on the nature of data point connections and the main approximation techniques. Alshammari notes that identifying the general equation structure simplified adding more algorithms to the framework. While constructing the table, the team identified gaps where new algorithms could fit. They successfully filled one by combining ideas from contrastive learning with image clustering, yielding an algorithm that classifies unlabeled images 8 percent better than a state-of-the-art baseline. They also demonstrated how a contrastive learning debiasing technique can boost clustering accuracy. The table’s design is flexible, allowing the addition of new rows and columns to accommodate novel types of data relationships. Hamilton suggests that I-Con encourages researchers to think creatively, fostering combinations of ideas they might not have previously considered. Overall, this elegant unifying equation, grounded in information science, covers a century of machine-learning research and opens many paths for future discoveries. Yair Weiss, a professor at Hebrew University of Jerusalem uninvolved with the study, praised I-Con as a rare example of unifying work that may inspire similar efforts across machine learning domains, especially important given the flood of new research papers each year. This research received support from the Air Force Artificial Intelligence Accelerator, the National Science Foundation AI Institute for Artificial Intelligence and Fundamental Interactions, and Quanta Computer.
Brief news summary
MIT researchers have created a unified "periodic table" of over 20 classical machine-learning algorithms using a new mathematical framework called information contrastive learning (I-Con). This framework shows that many algorithms function by minimizing differences between predicted and actual relationships in data. By categorizing algorithms based on these relationships and their approximation methods, the table not only organizes existing techniques but also uncovers new possibilities for algorithm development. A significant result of this work is a novel image-classification method that combines contrastive learning with clustering, leading to an 8% accuracy improvement over top models. Led by MIT graduate Shaden Alshammari and collaborators from MIT and Google AI, the research was presented at the International Conference on Learning Representations and supported by the Air Force and NSF. This unified view of a century’s worth of machine-learning progress is expected to accelerate AI advancements by promoting systematic innovation and reducing redundant efforts across diverse fields.
AI-powered Lead Generation in Social Media
and Search Engines
Let AI take control and automatically generate leads for you!
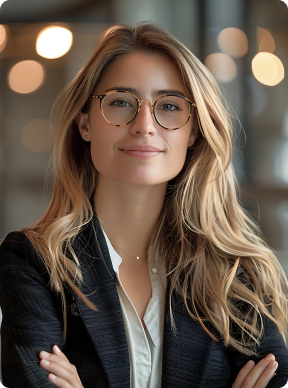
I'm your Content Manager, ready to handle your first test assignment
Learn how AI can help your business.
Let’s talk!
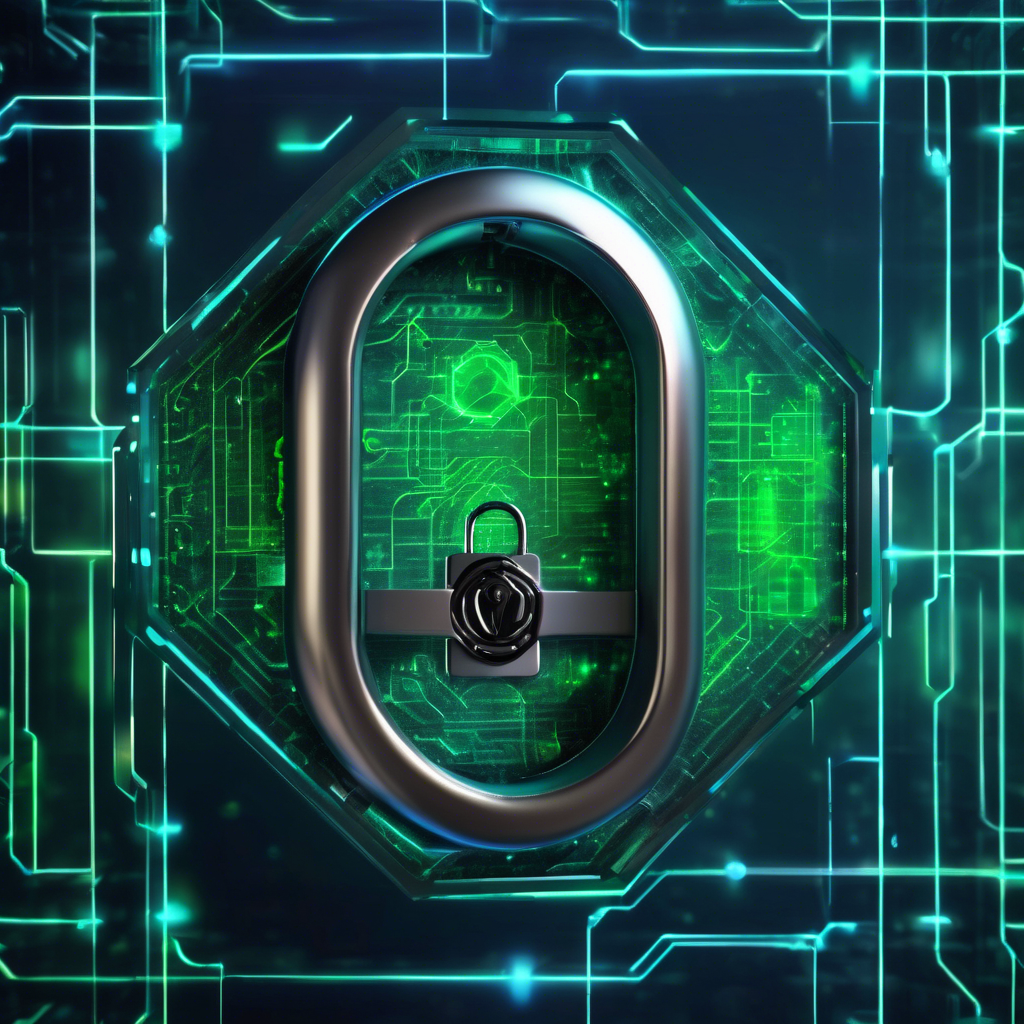
Privacy and Blockchain: Enhancing Security and Co…
Privacy and blockchain technology intersect in intriguing ways, mainly through advanced cryptographic techniques aimed at enhancing security and confidentiality for users.
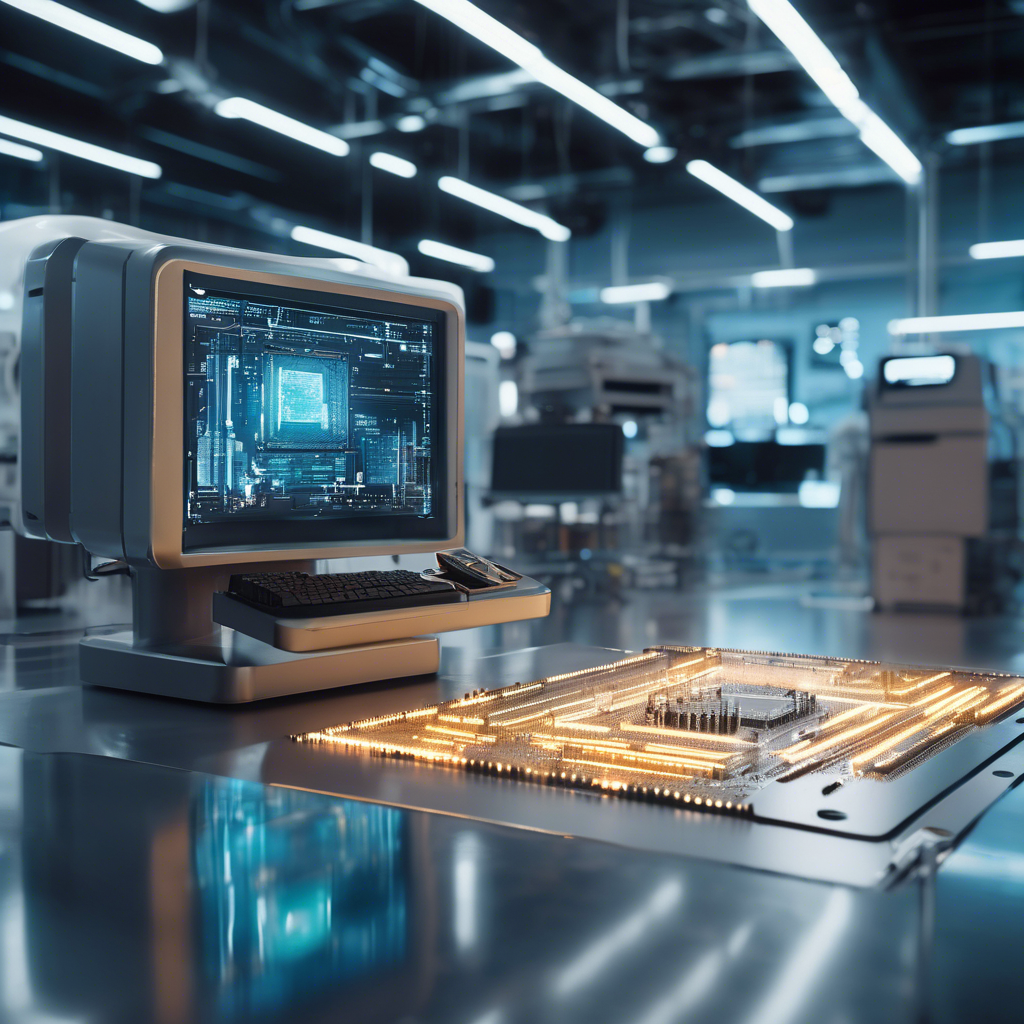
Trump to change controversial Biden-era restricti…
President Donald Trump plans to rescind Biden-era restrictions designed to prevent advanced technology from reaching foreign adversaries—measures criticized by major tech companies.
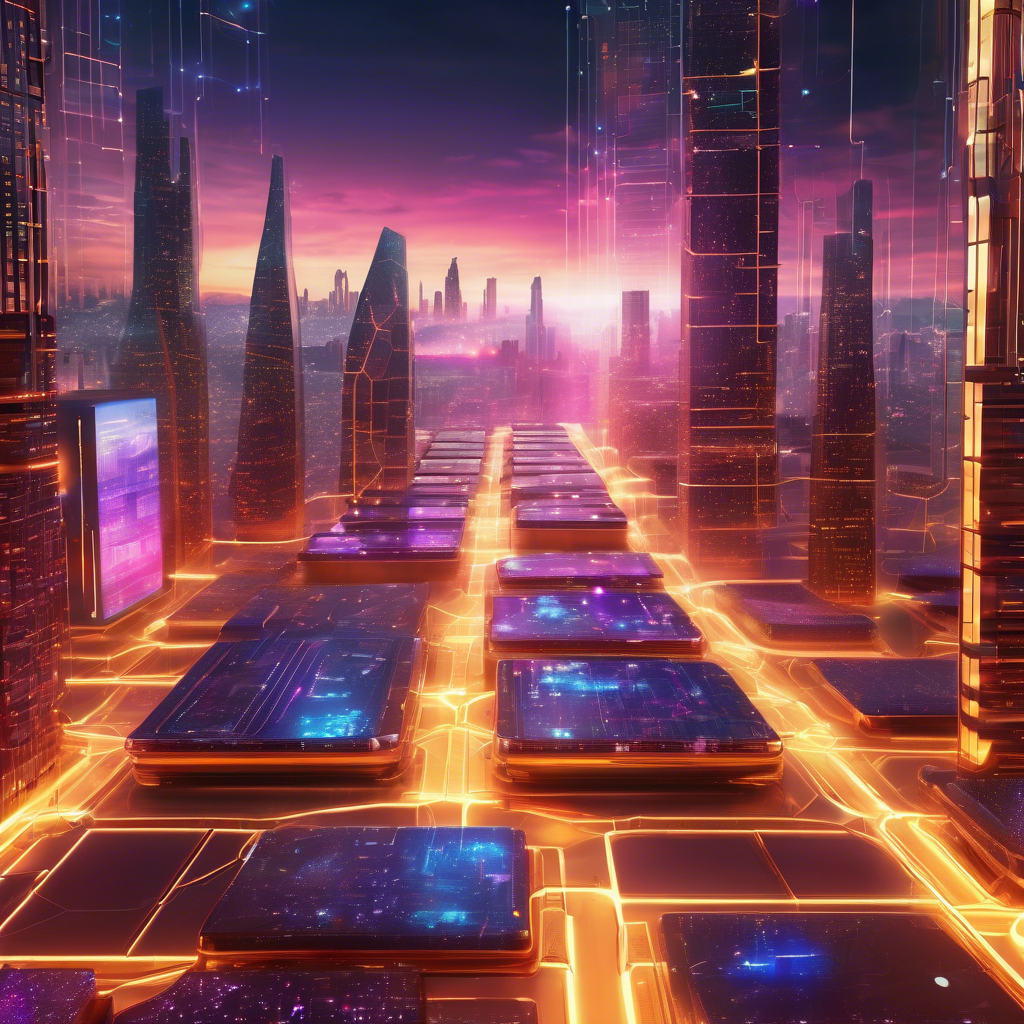
Blockchain Government Presents a $791.5 Billion M…
The global Blockchain Government market is rapidly expanding, valued at US$22.5 billion in 2024 and projected to soar to US$791.5 billion by 2030, with a robust Compound Annual Growth Rate (CAGR) of 81% from 2024 to 2030.
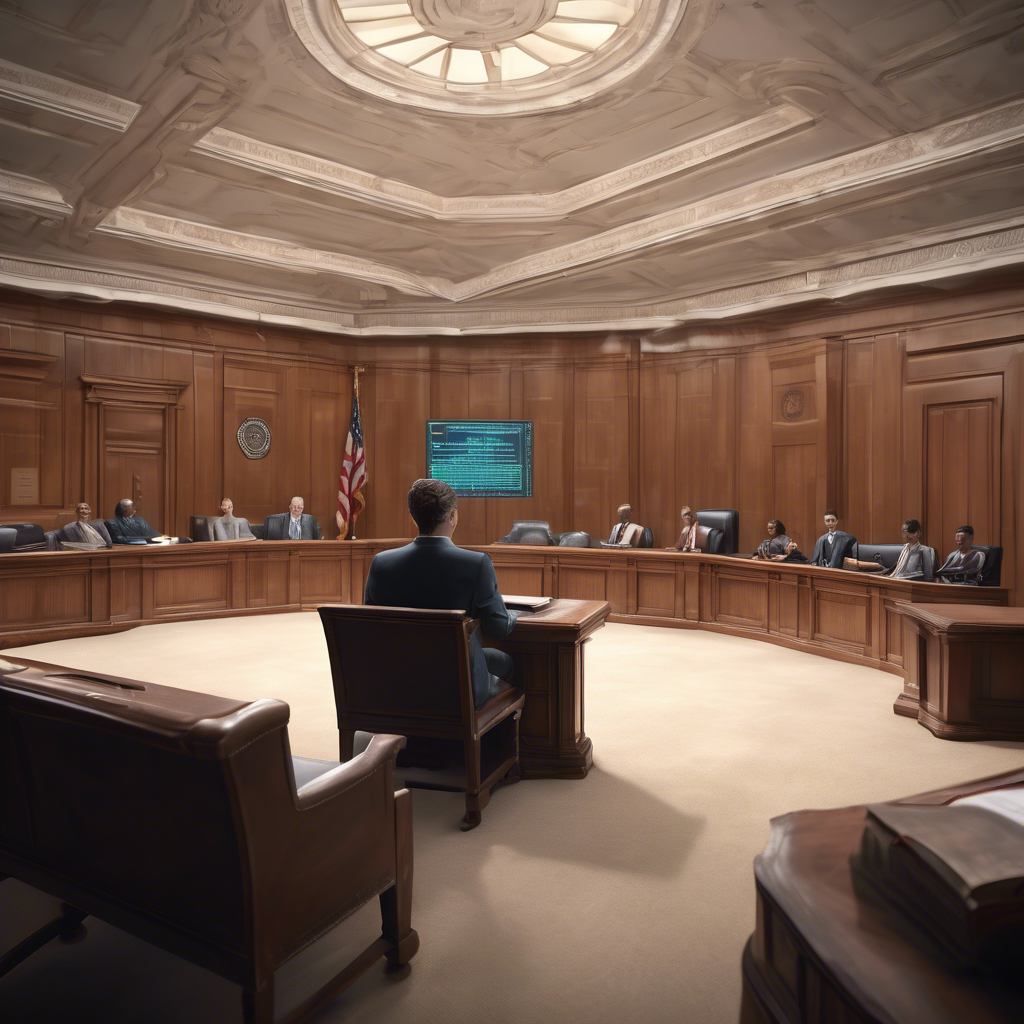
Man murdered in 2021 "speaks" at killer's sentenc…
In a pioneering move in U.S. courts, the family of Chris Pelkey, a man killed in a 2021 Arizona road rage incident, used artificial intelligence (AI) to create a video of him delivering a victim impact statement at his killer’s sentencing hearing.
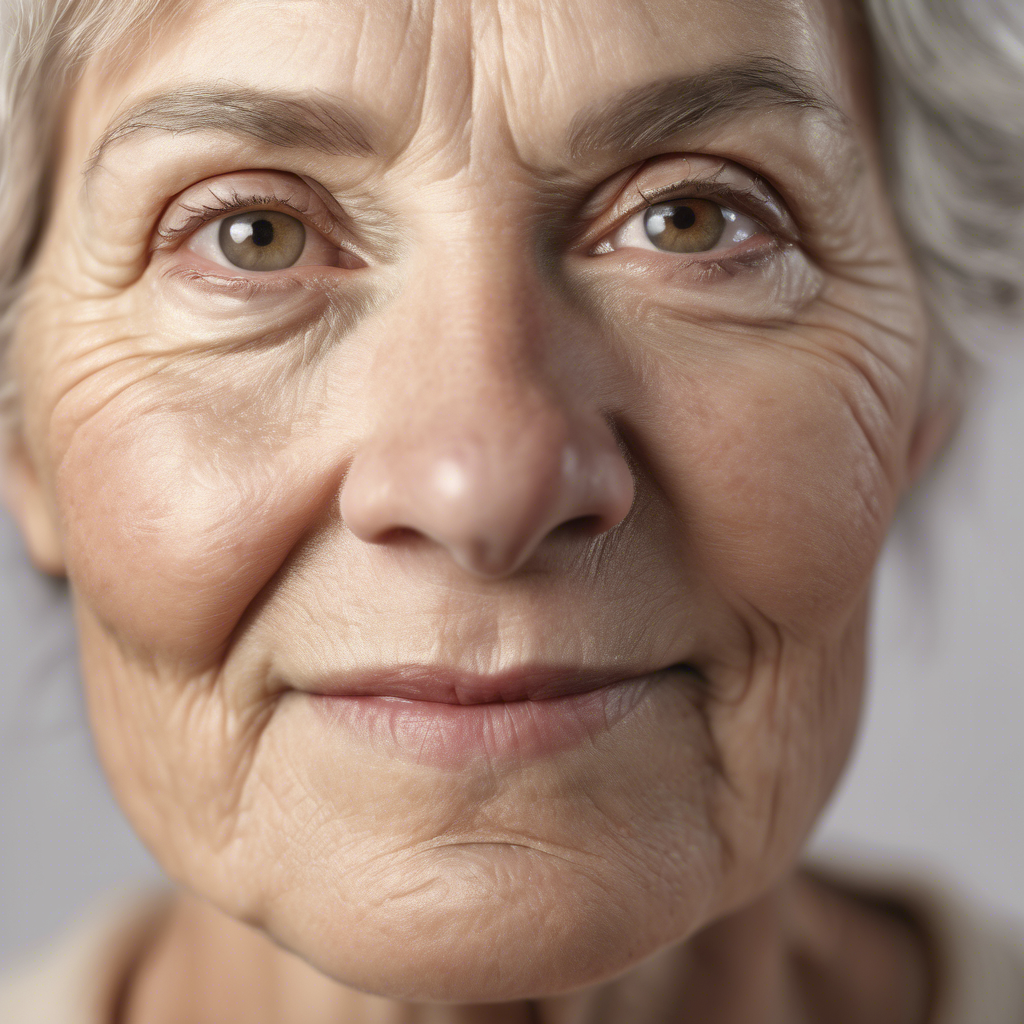
I Tried to See How I'll Age Using AI. It Wasn't a…
There’s nothing more attractive than someone who embraces their age gracefully.
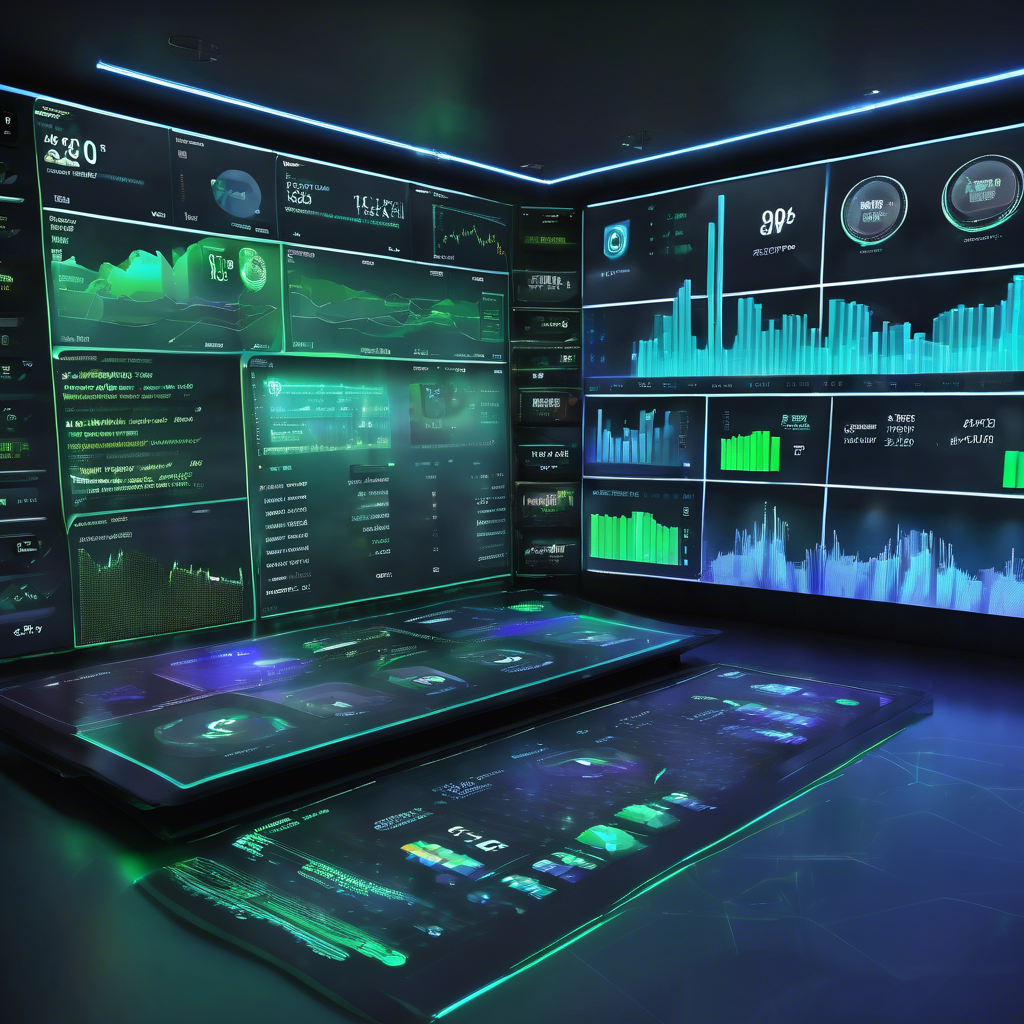
Crypto Market Roundup for May 5, 2025
On May 5, 2025, the global cryptocurrency market experienced a slight pullback, with the total market capitalization at $2.94 trillion, reflecting a 1.3% decrease over the past 24 hours.
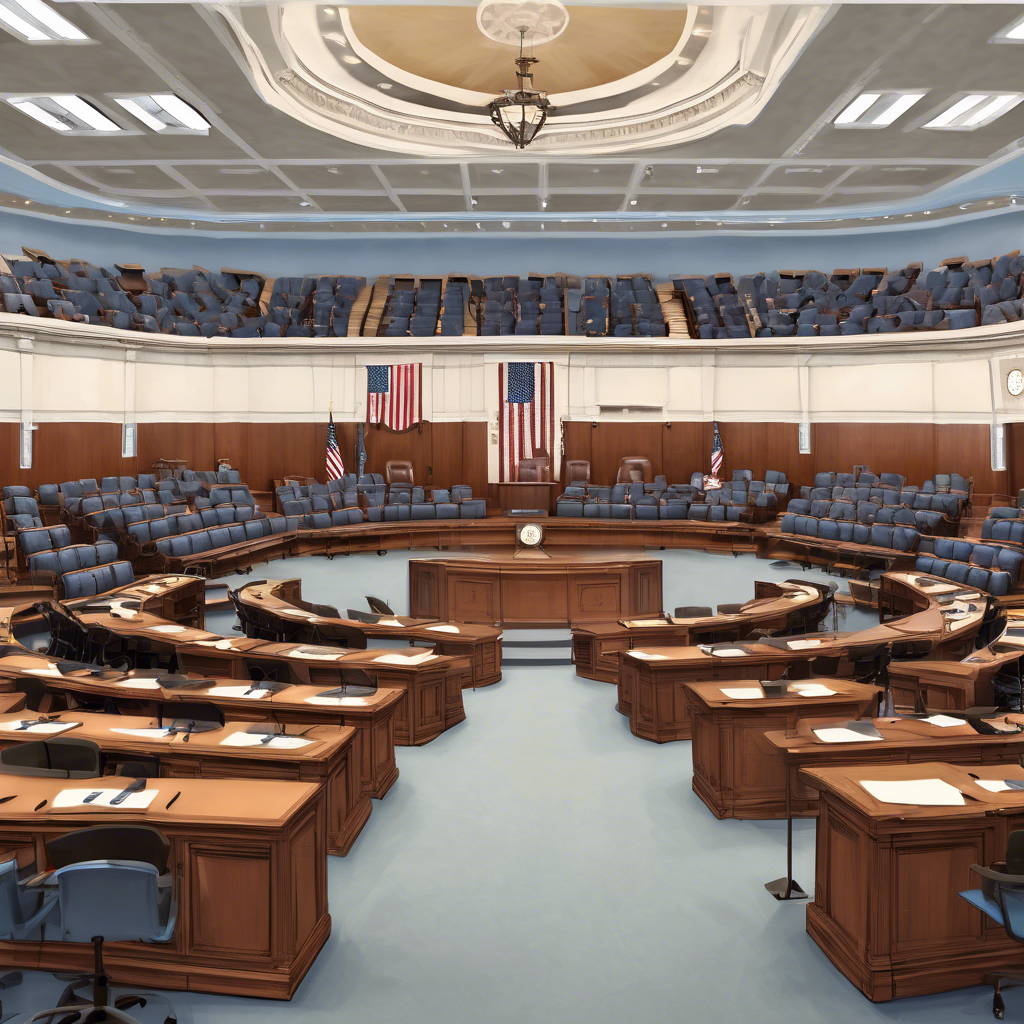
OpenAI CEO Sam Altman and other US tech leaders t…
On May 8, 2025, the U.S. Senate held a significant hearing focused on the transformative potential and global competition surrounding artificial intelligence (AI).